4 choses essentielles à rechercher dans une solution de détection des fraudes
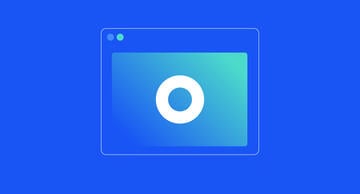
Pour votre entreprise, trouver la meilleure solution de détection et de prévention des fraudes peut s'avérer difficile. Les exigences des parties intéressées en interne et les fiches d’information des fournisseurs peuvent donner l’impression désolante que votre solution doit tout avoir et même plus. En réalité, votre choix doit simplement se porter sur un outil qui comporte tous les aspects que vous jugez indispensables et qui réponde aux besoins de votre entreprise. Cet outil doit contenir la plupart des fonctionnalités nécessaires prêtes à l’emploi, afin de réduire au minimum les personnalisations qui prennent du temps et des ressources.
Que doit offrir un outil de lutte contre la fraude pour répondre à vos besoins ? Pour commencer, une solution idéale doit pouvoir identifier et répondre à un large éventail de scénarios de fraude, qu’ils soient connus du secteur ou propres à votre entreprise. Cependant, il est également essentiel que l’outil soit en mesure de réagir à des cas de fraude inconnus et peut-être surprenants. Il doit offrir un ensemble de fonctionnalités polyvalentes permettant de collecter et d’analyser les données, de tirer des conclusions correctes, de prendre des mesures en fonction des résultats et, enfin, de produire des rapports complets. Cet outil devrait pouvoir s’intégrer dans votre écosystème actuel et, à un moment donné, il devrait devenir un atout dont votre équipe de fraude ne pourrait plus se passer.
Il est évident que c’est beaucoup demander à un logiciel de détection des fraudes. Toutes les solutions de détection des fraudes sur le marché n'étant pas à la hauteur de ces attentes, il est donc crucial que les entreprises fassent leurs recherches et trouvent un outil capable de fournir une surveillance complète des fraudes.
Pour vous aider à évaluer les principales exigences, notre Guide de l’acheteur pour l’évaluation des outils de détection des fraudes explique les neuf principales fonctionnalités qu’un outil de lutte contre la fraude doit réunir pour répondre aux besoins des institutions financières d'aujourd'hui. Voici un aperçu de quatre des neuf principales fonctionnalités que nous vous recommandons d’évaluer.
Fonctions clés d’un outil de détection des fraudes
1. Détecter un plus large éventail de fraudes en combinant l’apprentissage automatique avec un moteur de règles avancé.
Un moteur de règles avancé doté d’un ensemble de règles approprié filtrera les cas d'utilisation frauduleuse répondant à des critères spécifiques. Par exemple, le moteur de règles détectera les transactions dont l’heure, le lieu ou les montants sont en décalage avec un cas d'utilisation normal. Il peut également aider à détecter des cas plus complexes, comme les attaques d'hameçonnage ou les transactions sur des comptes de mules financières. Imaginez-le comme un système de filtres qui bloque les transferts, les anticipe ou alerte le système pour une authentification renforcée.
Toutefois, votre solution ne doit pas reposer uniquement sur des règles. Un système basé sur des règles ne peut plus rivaliser avec les attaques de fraude qui gagnent en complexité, en rapidité et en automatisation. Les bibliothèques de règles ne cessent de se développer, ce qui met le système sous pression, ralentit les opérations et augmente le taux de faux positifs. Afin de disposer des meilleures fonctionnalités pour combattre un large éventail de tentatives de fraude sans porter atteinte à la vitesse de traitement, pensez à une combinaison de règles avec des algorithmes d’apprentissage automatique.
L’apprentissage automatique est à la hauteur des attentes. Doté de la capacité d’analyser d'immenses quantités et variétés de données, il est un élément indispensable de votre dispositif de prévention et de détection des fraudes. Il peut facilement tirer parti des données avec peu d’intervention humaine.
Optez pour une solution d’apprentissage automatique qui met en œuvre différents algorithmes et, avec l’aide des experts de votre fournisseur, choisissez l’algorithme le mieux adapté à votre cas. Recherchez une solution d’apprentissage automatique qui vous donnera un aperçu du processus d’analyse et vous permettra de savoir pourquoi une transaction a été refusée ou acceptée.
2. Prévenir la fraude dès le départ.
Vous devez attendre de votre outil anti-fraude qu'il soit capable de détecter la fraude dès le départ. Veillez à ce qu’il réponde à vos exigences en matière de continuité des activités et, par conséquent, à ce qu'il prenne facilement le relais sur vos processus de fraude existants. Vous ne pouvez pas vous permettre de relâcher vos efforts de lutte contre la fraude et d'analyse des risques. Il est donc important de trouver une solution qui fournira un niveau de protection suffisant dès le premier jour. Vous devez disposer d’un système clés en main pour analyser les transactions en combinant un moteur de règles et l’apprentissage automatique. Les deux devraient fonctionner au déploiement, même sans données de référence.
De toute évidence, si la solution prête à l’emploi est un bon début, elle doit être suffisamment souple pour s’adapter à vos propres besoins et données.
- Détecter un plus large éventail de fraudes en combinant l’apprentissage automatique avec un moteur de règles avancé.
- Prévenir la fraude dès le départ.
- Appliquer une approche dynamique à vos flux d’authentification.
- Être prêt à relever les défis propres au canal mobile et exploiter tout le potentiel des données.
- ...
3. Appliquer une approche dynamique à vos flux d’authentification.
Le cadre de surveillance des fraudes devrait pouvoir s’intégrer aux options d’authentification multi-facteurs actuelles et futures. Il devrait évaluer en permanence le risque d’un événement particulier et, sur la base de cette évaluation, orchestrer le flux d’authentification. Il devrait déclencher de manière dynamique la méthode d’authentification la plus appropriée pour une situation donnée, en fonction de son niveau de risque. Par exemple, si une transaction est jugée suspecte, en raison d'un horaire inhabituel, de la localisation de l’utilisateur ou d’un montant sensiblement plus élevé qu’auparavant, votre solution devrait pouvoir renforcer les critères d’authentification au lieu de simplement rejeter la transaction ou de la mettre en attente pour un examen manuel.
4. Être prêt à relever les défis propres au canal mobile et exploiter tout le potentiel des données.
Le canal mobile pose des difficultés supplémentaires qui le distinguent de l’expérience bancaire standard sur Internet. Votre solution de lutte contre la fraude doit tenir compte de ces différences.
La surveillance du canal mobile doit tenir compte, entre autres, de la diversité des appareils et des systèmes d’exploitation ou du fait qu’aucun contrôle n’est exercé sur ce qui est installé sur ces appareils. S'il ne reconnaît pas les spécificités du canal mobile, l’outil risque de ne pas collecter tous les points de données et donc de tirer des conclusions erronées. Étant donné que les téléphones mobiles fournissent en général un contexte beaucoup plus riche et permettent une analyse plus avancée, il est essentiel de tirer parti du contexte plus large du canal mobile pour lutter contre la fraude mobile.
Votre cadre de surveillance des fraudes doit fournir une analyse basée sur un large éventail de données collectées à partir des appareils de vos utilisateurs. Ces données peuvent comprendre, par exemple, l’état de santé de l’appareil, en détectant, entre autres, si l’appareil a été débridé ou si une activité suspecte a été effectuée. Des informations peuvent également être fournies pour l’authentification et la biométrie, par exemple le score de reconnaissance faciale ou la force du code PIN. Les informations générales sur les appareils sont un autre exemple parmi un large éventail d’informations spécifiques aux téléphones portables, et peuvent inclure la version du système d’exploitation, le modèle de l’appareil, etc.
Ces points de données ne sont toutefois utiles que s'ils sont valables. Cela signifie que vous devez vous assurer que la collecte des données et le transfert entre l’appareil mobile et le serveur sont sécurisés. Un canal de communication sécurisé indépendant des autres protocoles de communication existants garantira la fiabilité de l’état de sécurité de l’appareil dès son arrivée dans votre système de surveillance des fraudes.
Exigences clés supplémentaires pour votre évaluation
Le but ultime d’un cadre de lutte contre la fraude est de mettre fin aux activités criminelles tout en rationalisant les activités légitimes. Les outils simples ne suffisent plus. La fraude est simplement en constante évolution parce qu’elle présente un énorme potentiel de profit pour les criminels ; c’est pourquoi vos armes anti-fraude doivent elles aussi évoluer.
Téléchargez le Guide de l’acheteur pour l’évaluation des outils de détection des fraudes afin de connaître les neuf principales exigences pour une solution moderne et efficace de lutte contre les fraudes, de l’apprentissage automatique à la capacité d’orchestrer les flux d’authentification.
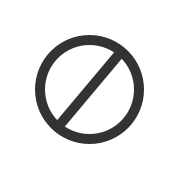